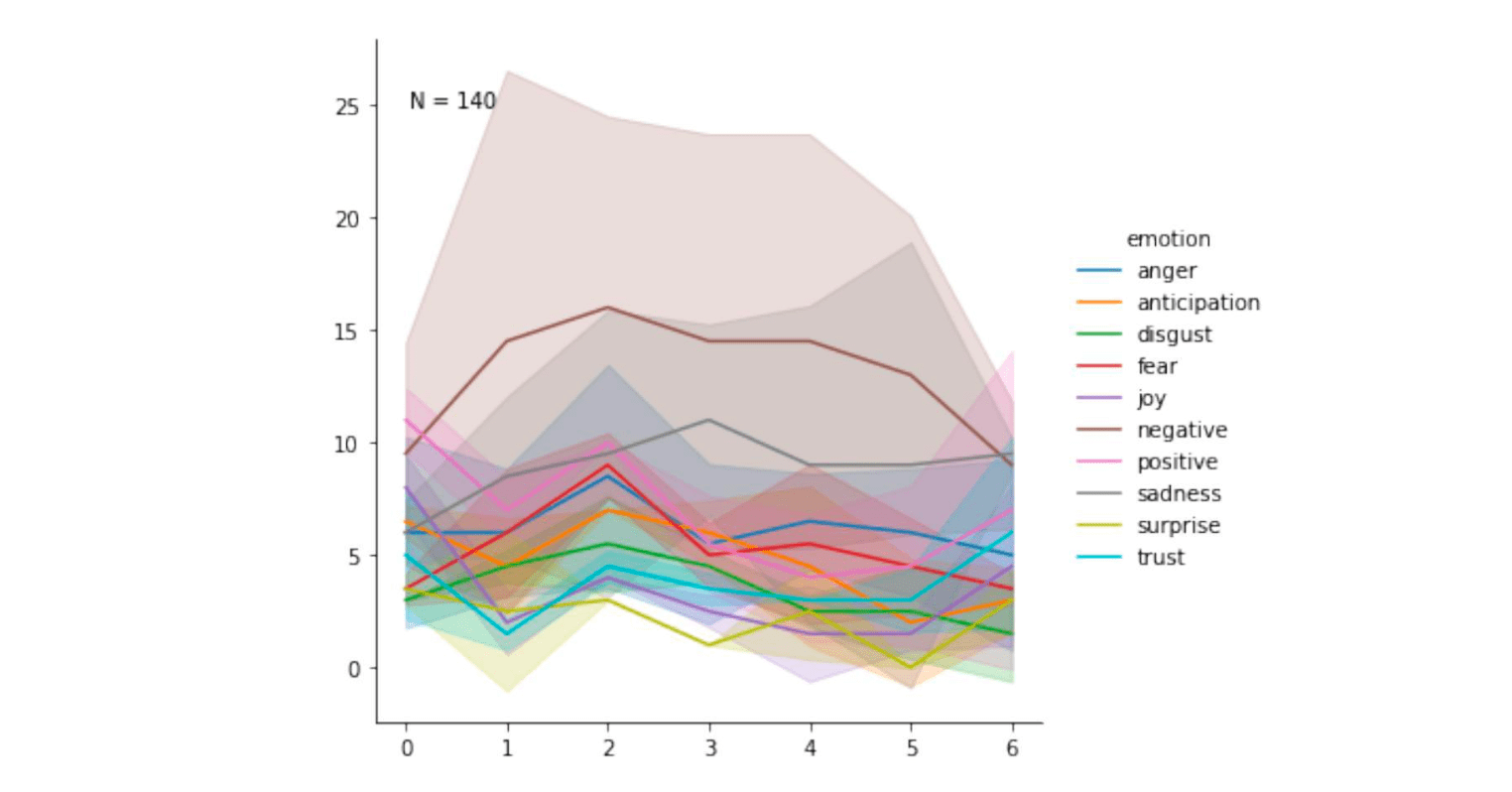
Storytelling comes naturally to humans, but is exceptionally difficult for artificial intelligence. Machine learning models that generate remarkably fluent text still struggle to craft narrative arcs spanning paragraphs or pages. New research from Stanford University demonstrates how "emotion maps" could improve story generation.
The key challenge is imbuing AI systems with a high-level understanding of plot and long-range dependencies - core elements of compelling stories. Without such top-down guidance, machine-written tales easily become repetitive and disjointed.
The Stanford project explores a technique called hierarchical generation. This involves first creating a short premise or prompt, then expanding that outline into a full story. The premise acts like an anchor, guiding the system to remain on topic and logically progress the narrative.
But how can we represent a good premise for AI? The researchers move beyond using text, instead generating "emotion maps." These maps contain a series of numerical scores representing different emotive attributes. Each score tracks how positive or negative, sad or joyful consecutive sections of the story feel.
For instance, a map may start very positive, then become sadder, and end on a more hopeful note. Feeding these maps as prompts produces stories that logically follow the intended emotional arc. The numbers offer a bird's-eye view of the narrative's affective flow.
Remarkably, this numerically-conditioned approach achieved comparable results on two standard story datasets as previous efforts using text prompts. The generated stories displayed coherent grammar and punctuation, sensibly reacting to the emotion map's ups and downs.
To better understand the relationship between maps and stories, the researchers introduced a new metric called Average Emotional Similarity. This quantifies how closely a story's actual emotion aligns with its prompt map. Initial results demonstrate some correlation, confirming the maps exert influence on the tone of generated text.
There are several advantages to conditioning story generation on simplified cues rather than verbose outlines. Maps neatly capture narrative essence in a compact, rapidly computed form. Reducing hand-authoring effort also enables building larger training datasets.
However, many challenges remain. Emotionless stories often flummox the system, producing bizarre outputs. Repetition and hallucination still crop up, demonstrating the need for greater plot coherency. And evaluating story quality continues to prove difficult without extensive human judgement.
Nonetheless, this research highlights the potential of hierarchical methods to imbue AI storytelling with greater purpose. The raw material exists in today's pretrained language models - machines that have "read" vast amounts of text. We must guide them toward higher reasoning about concepts like theme, characters, and dramatic structure.
Interactive tools could empower human authors to easily craft emotion maps, generating stories tailored to their creative vision. Teachers might build maps to help students practice writing logically paced narratives. Therapists could use emotive cadences to gently evoke memories or feelings from patients.
The capacity for machines to conjure compelling tales could transform how we communicate ideas and experiences. But achieving this dream will hinge on passing down our innate sense for what makes a story worth telling. With innovations like emotion maps lighting the way, artificial authors inch toward unlocking our imagination.
source:
Hierarchical, Feature-Based Text Generation