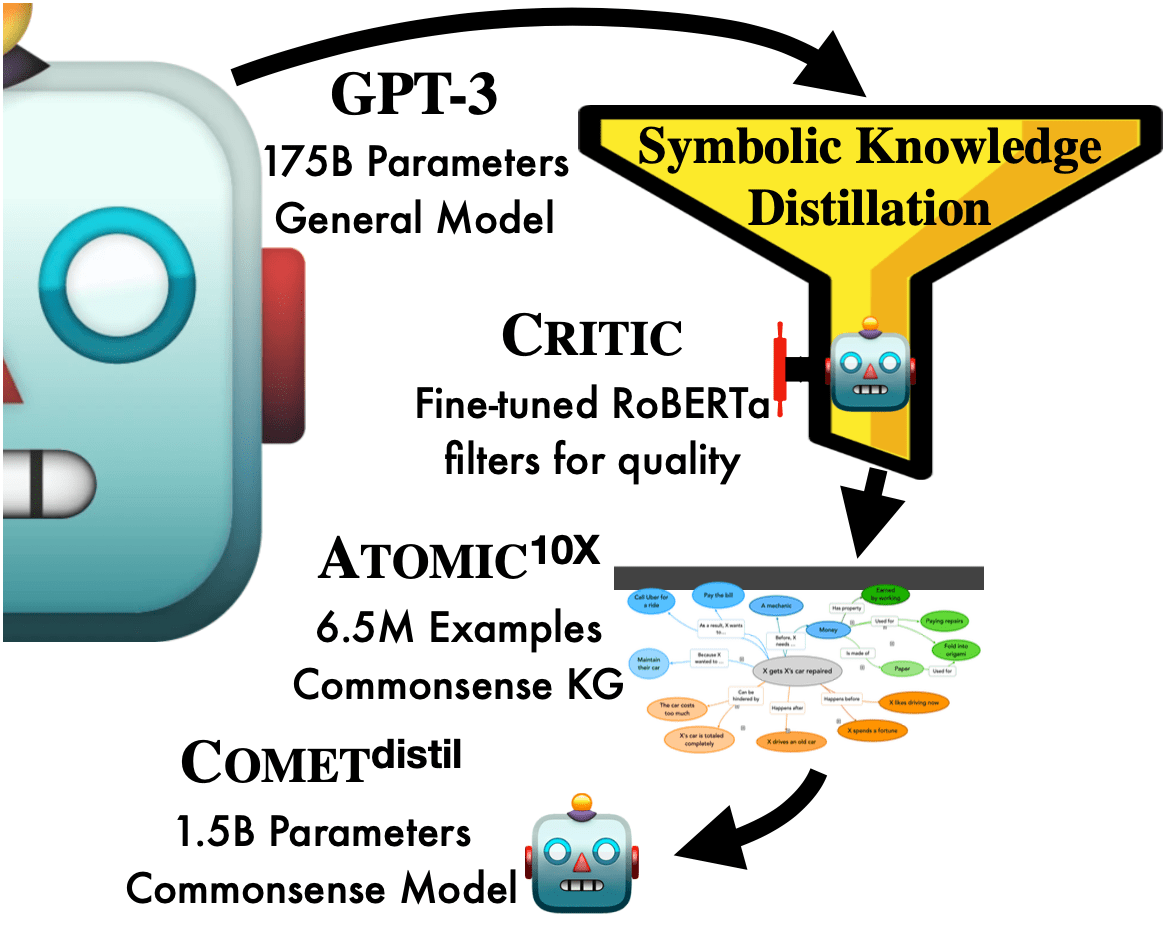
Artificial intelligence (AI) systems still lack true understanding of the world and rely heavily on training data provided by humans. An ongoing challenge is developing AI with more generalized common sense - basic knowledge about how the world works that humans acquire through experience.
Researchers have proposed compiling common sense into knowledge graphs - structured collections of facts. But these require extensive manual effort to create and often have gaps. Now, scientists at the University of Washington and the Allen Institute for AI have demonstrated a new technique called "symbolic knowledge distillation" that automates common sense acquisition for AI. Their method transfers knowledge from a large, general AI model into a specialized common sense model, without direct human authoring.
The researchers used GPT-3, a leading natural language AI model from OpenAI, as the knowledge source. GPT-3 was prompted to generate common sense inferences about everyday scenarios, creating a knowledge graph called ATOMIC10x with 10 times more entries than human-authored versions. This automatic approach achieved greater scale and diversity of common sense than manual authoring.
To improve the accuracy of the AI-generated knowledge, the researchers trained a separate "critic" model to filter out incorrect inferences. With this critic, ATOMIC10x attained over 96% accuracy in human evaluations, surpassing 86.8% for human-authored graphs. The knowledge graph both exceeded humans in quantity and matched quality.
The researchers then trained a compact common sense model called COMET on the ATOMIC10x graph. Remarkably, this smaller COMET model outperformed its massive GPT-3 teacher in generating accurate common sense inferences. It also improved on models trained with human-written knowledge graphs.
This demonstrates an alternative pipeline - from machine-generated data to specialized AI models - that can exceed human capabilities for common sense acquisition. The researchers propose that humans can play a more focused role as critics, rather than manually authoring entire knowledge bases.
The new distillation technique paves the way for more capable AI assistants, chatbots, and robots that understand implicit rules of everyday situations. Common sense helps AI converse naturally, perform physical tasks, and make logical inferences about causality and human behavior. Automating common sense at scale remains a grand challenge for human-like artificial intelligence.
This research exemplifies how large AI models like GPT-3 can transfer knowledge to more specialized applications through automatic generation. While general models have limitations in narrowly defined tasks, their broad learning makes them valuable teachers. Distillation techniques focus that broad knowledge into optimized models for specific needs like common sense.
Business leaders should track such advances that make AI more generally capable and useful across applications. Automating the acquisition of common sense can complement training data curated by humans, reducing manual bottlenecks. AI models endowed with common sense hold promise for everything from chatbots to autonomous systems to creative applications. While current methods are imperfect, rapid progress is being made - foreshadowing AI assistants that understand the world more like we do.
Sources:
Symbolic Knowledge Distillation: from General Language Models to Commonsense Models