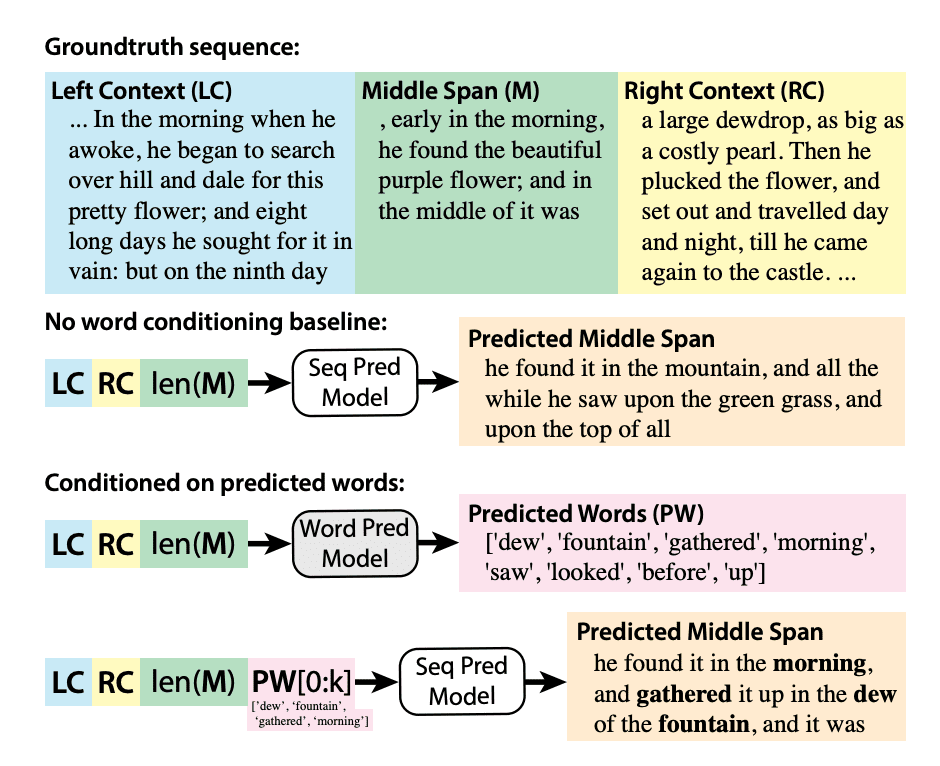
Stories unfold step-by-step, but writers sometimes get stuck on how to connect one part to the next. AI research from 2019 explored how to automatically generate reasonable suggestions for missing sections of text. This "story infilling" aimed to assist creative writing by proposing ideas that align with the existing story while still surprising the author.
The researchers found that standard AI language models at the time struggled to balance coherence with novelty when filling in gaps. The generated text ended up too boring or too random. To address this limitation, they designed a two-step hierarchical system:
- First, the AI randomly selected a few rare, interesting words that could plausibly fit into the storyline based on the context. For a medieval fantasy passage, it might suggest words like "dragon," "princess," or "castle." The system focused on rare words since they provide more information to guide the rest of the text.
- Second, the system generated full sentences conditioned on those interesting words, searching likely combinations that form coherent text. Leveraging the rare words prevented repetitive suggestions, while allowing the model to focus on fluency and coherence.
The researchers tested story infilling on passages from children's tales with missing sections of 15-30 words. Human evaluators preferred the hierarchical model's suggestions over non-hierarchical methods, which sacrificed diversity or quality.
The field has greatly advanced since 2019 with models like Claude and GPT-4. Yet even powerful AI still struggles with high-level plot and character consistency. Explicitly decomposing generation into steps of planning and drafting, as humans do, is one way to address these challenges. While AI cannot replace human creativity, structured models could soon provide useful brainstorming and revision tools for real authors.
Sources:
Unsupervised Hierarchical Story Infilling