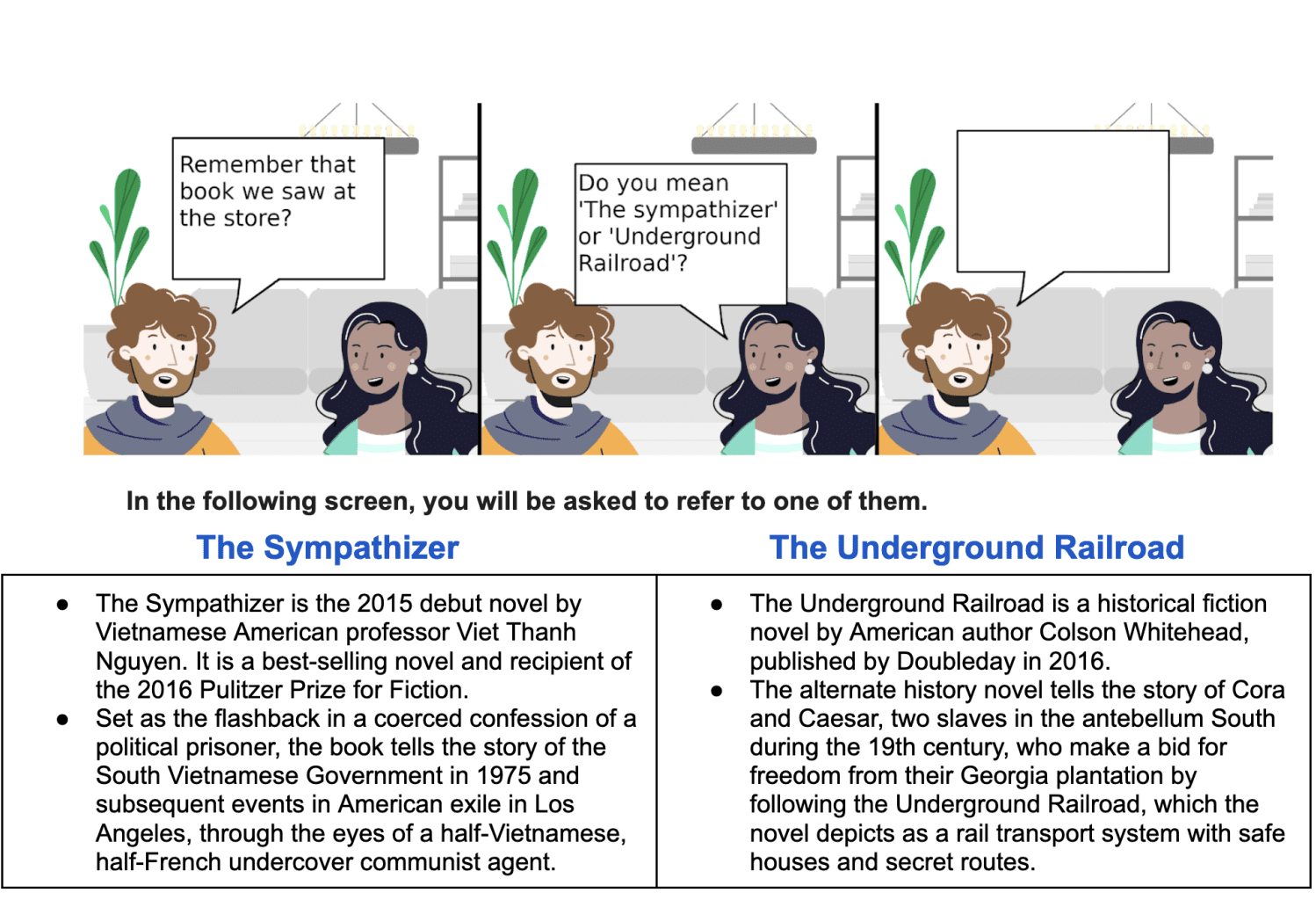
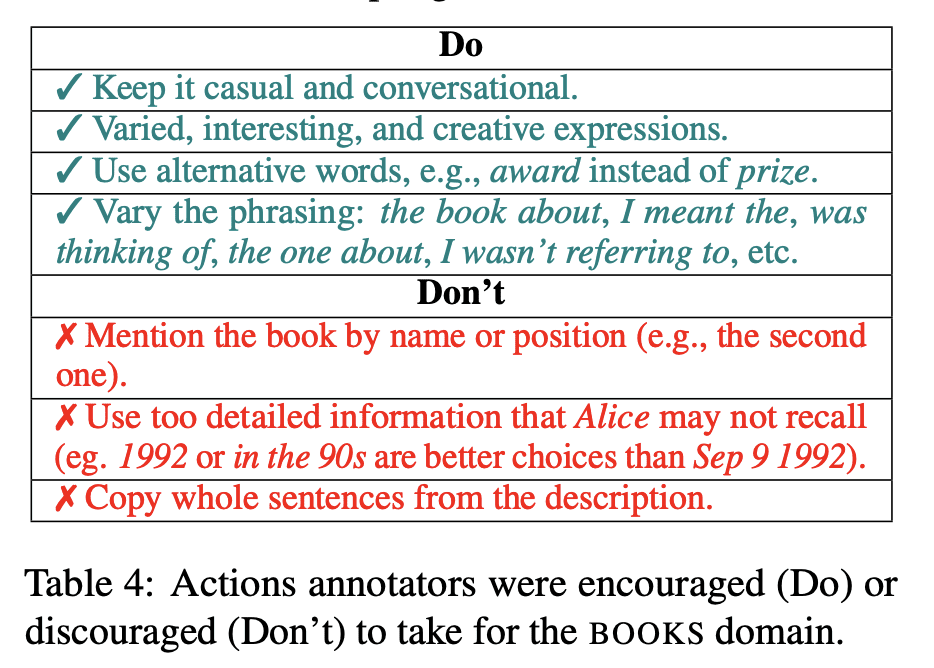
Artificial intelligence (AI) has made great strides in recent years, with systems able to hold conversations, answer questions, and make recommendations. However, these systems still struggle with the subtle complexities of natural human language. In particular, when people are choosing between options, they often refer indirectly to their choice rather than using the exact name. For example, when asked "Do you want the chocolate or vanilla ice cream?" someone may respond "I'll have the darker one" rather than saying "chocolate." Teaching AI systems to understand such indirect references is an important next step to make interactions feel more natural.
Researchers at Google have developed a new dataset and models to tackle this problem, summarized in a recent paper. Their key innovation was creating a cartoon-style interface to collect natural conversational responses from regular people choosing between two options, such as recipes, books or songs. By framing it as a casual chat between friends looking back on options, they encouraged indirect references like "the one with the green cover" or "the sweeter dessert" rather than using item names directly.
After collecting a dataset of over 40,000 such indirect references across three categories, they tested different AI models at picking the intended option based on the reference. With no background knowledge beyond the item names, accuracy was just above random guessing. But given relevant textual descriptions of each item, accuracy reached over 80% with the best models. This is promising compared to previous results, but still leaves room for improvement to handle more subtle references.
The researchers also showed the models can learn general patterns that transfer between categories, rather than just memorizing item-specific clues. So training on books, songs and recipes enabled reasonably good performance on each area without needing new training data. This is important for applying the technology efficiently to new domains.
For business leaders, this research highlights both the progress and remaining challenges in making AI conversational interfaces feel natural. Indirect references are common in human conversations, so handling them well is key to users' comfort with AI systems. These results suggest current AI capabilities could support basic back-and-forth interactions, but with some limitations.
Looking ahead, there are several opportunities to build on this work:
- Expanding training data to cover more domains, languages and cultural references would make systems more robust.
- Exploring different input modes beyond text, like images, audio and video, could improve understanding of indirect references.
- Better reasoning capabilities would allow AI systems to make inferences about items, rather than relying completely on background knowledge descriptions.
- Retrieval augmented models that proactively gather relevant information could improve disambiguation with limited initial knowledge.
- Decomposing complex references into simpler concepts could enable understanding of indirect comparisons like "the happier song."
As conversational systems become integrated into more products and workflows, demand will grow for smooth and natural interactions. Investing in AI advances that unlock more human-like language understanding seems likely to offer strategic value across many industries. While current capabilities are promising, there is still plenty of work needed to truly reach the subtlety and flexibility of human conversation.
Sources
Resolving Indirect Referring Expressions for Entity Selection