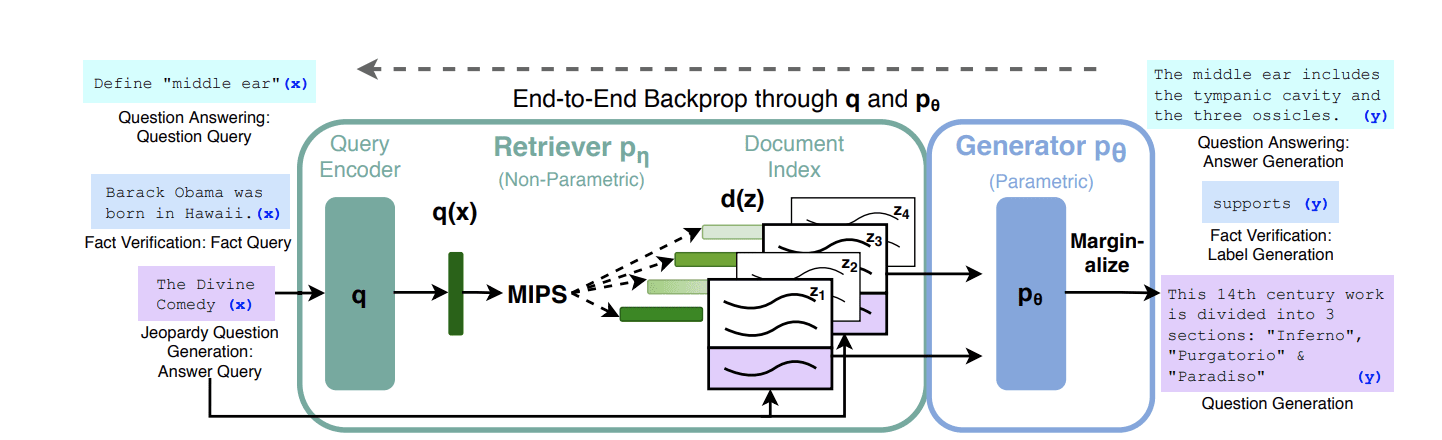
Artificial intelligence has made tremendous strides in recent years, with systems like ChatGPT able to carry on surprisingly cogent conversations. Yet most AI still struggles when it comes to generative tasks requiring real-world knowledge - providing informative answers to open-ended questions, for example, or writing articles on complex topics.
This is because most AI models rely solely on their internal 'parametric' memories - the knowledge encoded in their neural network parameters through training. While extensive, this knowledge is still limited compared to the breadth of human knowledge. Humans augment our memories with outside information sources - books, websites, experts - to reason about topics beyond our expertise.
A new class of AI systems aims to give models this same ability, combining parametric neural networks with 'non-parametric' memories - large external knowledge bases. Termed retrieval-augmented generation (RAG), these hybrid systems learn to retrieve and incorporate external knowledge when generating text.
A recent paper from Facebook AI Research explores RAG models in depth. Their RAG system combines a pre-trained parametric text generation model with a non-parametric memory of Wikipedia. The parametric component generates fluent text, while the non-parametric memory provides factual grounding.
Here's how it works:
- The system takes in a text input - a question, prompt, etc.
- A neural retriever module queries the Wikipedia index to find relevant articles.
- The retriever passes these articles to the text generator.
- The generator conditions on both the input text AND retrieved articles to produce an informative, grounded response.
This simple framework allows RAG models to achieve state-of-the-art performance across a variety of knowledge-intensive NLP tasks:
- Open-domain question answering - RAG models set new records on challenging QA benchmarks, with the flexibility to generate free-form answers.
- Fact verification - For detecting whether claims are supported or refuted by Wikipedia, RAG performs on par with complex pipeline systems.
- Abstractive question answering - RAG provides more specific, factual responses to open-ended questions compared to text generators without retrieval.
- Diverse, grounded text generation - On challenges like Jeopardy question generation, RAG outputs are substantially more diverse, specific and accurate.
RAG represents a milestone for AI knowledge and reasoning. By combining neural networks with external memory, these models enjoy the best of both worlds - the generalization of large pretrained models and the versatility of lookup-based reasoning.
The modular framework also makes RAG highly adaptable. The same overall architecture can be applied to any task, simply swapping in different parametric models or knowledge sources. And the external memory can be updated on the fly, allowing RAG systems to dynamically learn about new concepts or changes in the world.
For business leaders, RAG has profound implications. As AI grows more knowledgeable and reasoning-driven, it can automate higher-value workflows rather than just narrow, repetitive tasks. A RAG assistant could provide executives with synthesized briefings each morning about the latest news on their company and industry. Customer service representatives could rely on RAG systems to quickly provide accurate, customized responses to consumer questions. The same technology could even help knowledge workers be more productive at writing, research and analysis.
Of course, challenges remain. Knowledge bases like Wikipedia have biases and gaps that can propagate into model behavior. There are still limitations to neural networks' reasoning capacities even when augmented with retrieval. And there are open questions around how to effectively train and deploy RAG across settings.
But by learning to read before writing, this new breed of AI unlocks immense possibilities. RAG represents a promising path toward more intelligent, useful AI applications in business and beyond. The fusion of parametric and non-parametric memory illustrates how, like humans, artificial intelligence can accomplish more by working together with external knowledge.
Source: