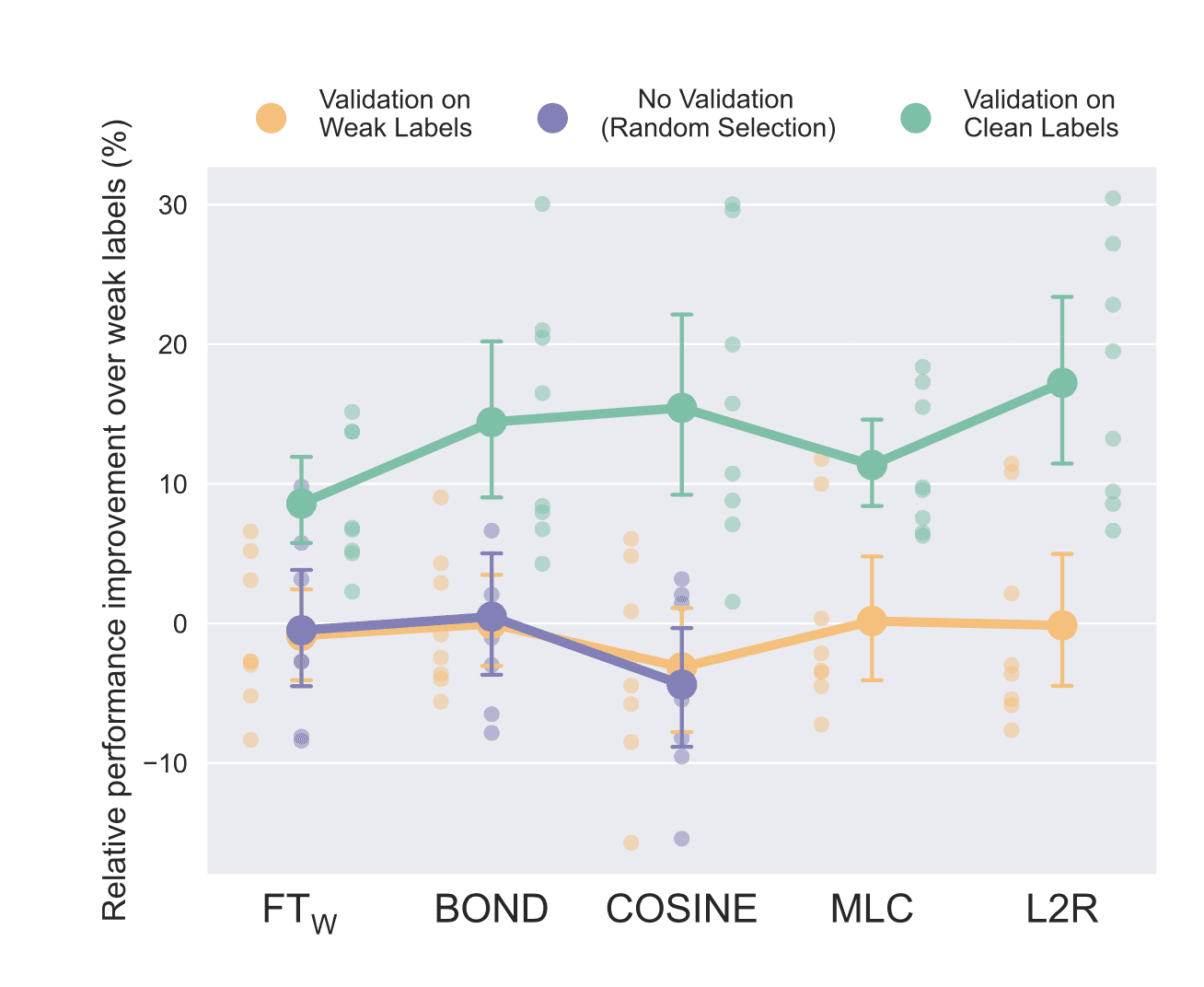
Weakly supervised learning is a popular technique in AI research that aims to train models using noisy, imperfect data instead of clean, human-labeled data. The goal is to alleviate the costly data annotation bottleneck. But new research suggests these methods may be significantly overstating their capabilities.
Researchers from Saarland University thoroughly evaluated several widely-used weakly supervised learning techniques on diverse natural language tasks. They found that with no clean data available, the methods completely failed to improve over basic weak labels. More concerning, commonly used clean validation data for model selection could be more efficiently leveraged by simply training models directly on it instead.
On benchmark datasets, sophisticated weakly supervised approaches were handily outperformed by fine-tuning models on as little as 5-10 clean examples per class. Even combining weak and limited clean data, a simple two-stage fine-tuning approach worked best. The purported benefits of complex weakly supervised methods largely vanished in realistic low-data situations.
The findings cast doubt on claims of progress in weak supervision and reveal flaws in how the techniques are evaluated. The reliance on sizable validation data underscores their limitations for real-world application. While weak supervision remains appealing for bypassing human labeling, current techniques seem to overpromise.
For business leaders, this research highlights pitfalls of trusting "state-of-the-art" AI before rigorously testing if it applies to your use case and data constraints. Clever weakly supervised approaches may not live up to claims on small datasets. But when designed and validated appropriately, they can still unlock unique value. Disillusionment with AI is often simply due to mismatch with practical realities. Aligning research with real-world needs is vital to deliver robust AI that businesses can confidently leverage.
Sources: