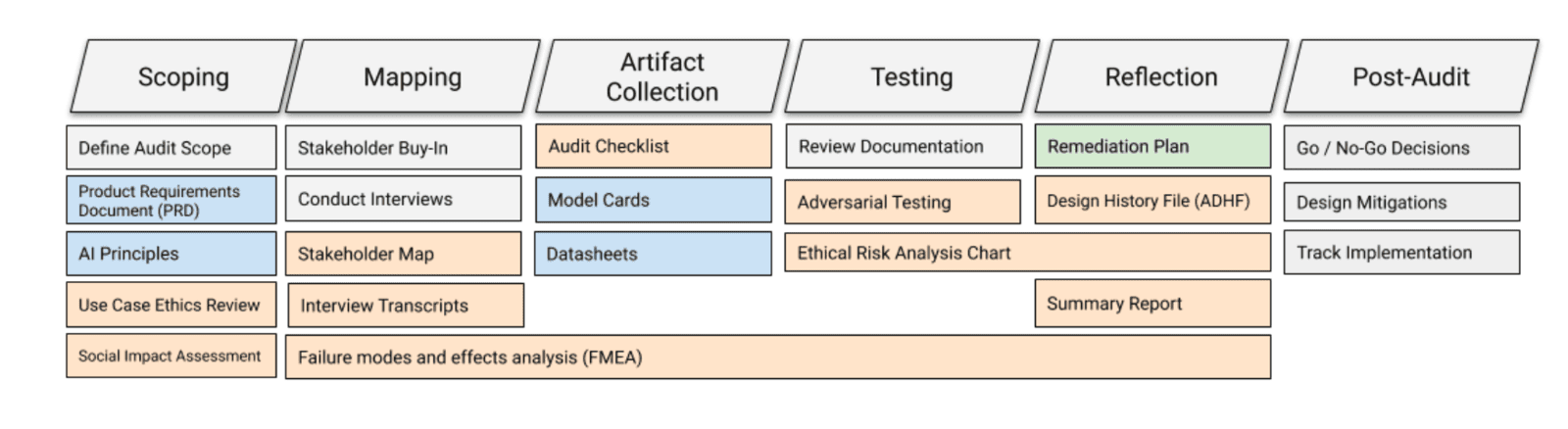
A research paper proposes formal internal audits as a mechanism for technology companies to ensure their artificial intelligence (AI) aligns with ethical priorities before deployment. Conducting rigorous self-assessments throughout development can make AI more accountable to society.
AI systems like facial recognition, predictive policing algorithms, and social media filters are increasingly affecting people's lives. However, external audits by journalists and academics often find these technologies disproportionately harm marginalized groups through biases encoded in training data or design choices.
By only auditing after deployment, it becomes difficult to fix issues rooted in early development stages. The authors argue that organizations creating AI should perform ongoing internal audits to catch problems sooner. This would complement external oversight.
Drawing inspiration from quality control practices in aerospace, medicine, and finance, the researchers outline a framework called SMACTR to structure internal AI audits. It comprises five stages:
- Scoping: Confirm the AI system's intended use case and principles it should uphold. Review potential social impacts.
- Mapping: Document the development process and people involved for traceability. Start a risk analysis.
- Artifact Collection: Gather key documents like model cards explaining model limitations and datasheets detailing training data.
- Testing: Evaluate risks through methods like adversarial attacks to fool models and surface failure cases.
- Reflection: Finalize the risk analysis. Develop a mitigation plan to address issues before launch.
At each stage, auditors generate artifacts like stakeholder maps, checklist procedures, and risk profiles. Together these produce an audit trail enabling organizations to validate if projects adhere to ethical objectives.
For example, an internal audit could evaluate a proposed income scoring algorithm to assess financial risk for lending decisions. The scoping stage would analyze how denying loans to "high-risk" individuals perpetuates inequality. Mapping would outline the engineering team and main model components. Artifact collection would include documentation of the training process and data sources. Testing would probe for biases like lower scores for racial minorities. The reflection would produce an action plan to address identified biases before deployment.
Unlike external audits focused on model outputs, internal assessment provides access to inside processes and data. The framework aims to translate abstract AI principles like fairness into concrete practices for responsible development.
The authors acknowledge limitations. Internal auditors share incentives with the audited organization, risking biased assessments. The framework also requires extensive documentation which slows rapid development. And ambiguity remains around acceptable thresholds for risks versus model accuracy and business objectives.
Nonetheless, the SMACTR methodology provides an initial structure for companies to audit algorithms against ethical priorities throughout the creation process, rather than just critiquing systems after launch. This proactive approach can uncover issues early when they are easier to fix, and prevent harmful technology from ever reaching users. The framework will require refinement, but represents a step towards aligning AI with societal values.
For business leaders, this research reinforces that ethical AI requires continuous review, not just post-deployment auditing. Companies should formalize auditing units with technical and ethical oversight separate from product teams. Following a standardized methodology can demonstrate accountability. Documentation and transparency will be key.
While external audits remain essential, supplementing these with rigorous internal assessments makes it likelier technologies empower society broadly rather than negatively impact vulnerable communities. As AI proliferates, adopting structured self-evaluations will help ensure businesses uphold their principles. The proposed framework offers a template for auditing AI with ethics in mind at every development stage.
Sources: