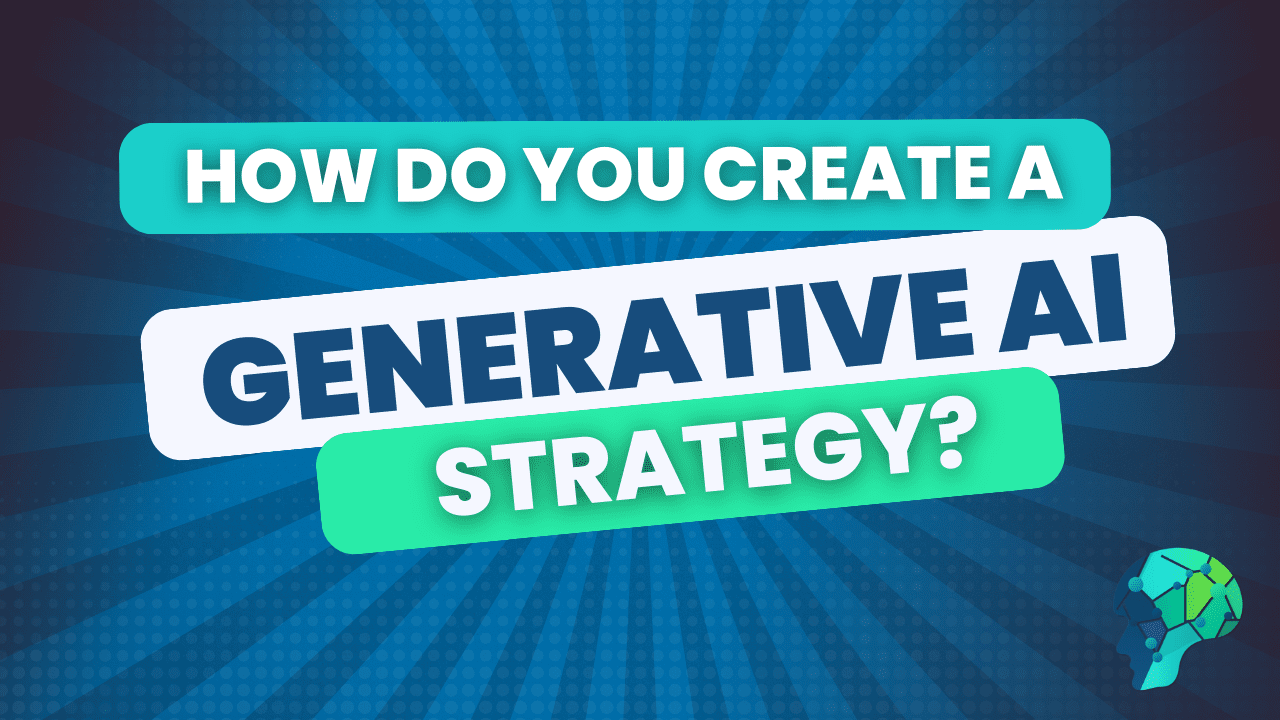
Generative artificial intelligence is one of the most promising and rapidly advancing technologies today. Systems like DALL-E 2, GPT-4, and Stable Diffusion showcase the enormous potential of generative AI to create novel and high-quality content based on text prompts.
As businesses realize the transformative capabilities of these systems, developing a sound strategy is crucial to harness generative AI successfully. An ill-thought-out approach risks wasted resources, unmet expectations, and public backlash over ethics or quality issues. On the other hand, a prudent strategy that aligns investments to core competencies can provide tremendous competitive advantage.
Here we offer practical guidance on crafting an effective generative AI strategy based on best practices. We cover critical considerations around technology evaluation, use case identification, model development, testing rigor, ethics review, and feedback loops that improve outputs continuously. The discussions aim to provide actionable advice that applies regardless of your company's size, industry, or current AI maturity level.
Assessing the Technology Landscape
The starting point in defining a generative AI strategy involves assessing the vendor and technology landscape to understand capabilities, limitations, and trade-offs.
Several vendors offer generative AI models today, including open-source options and commercial solutions. While capabilities grow rapidly, no single provider offers the best solution across all parameters. Factors like cost, ease of use, computational needs, output quality, allowed applications, content filtering, and privacy controls differ enormously between options. Spending resources to evaluate alternatives against your specific priorities is essential before committing to any platform or provider.
Additionally, while much attention goes to Ai foundations model offerings, your strategy may benefit from assessing complementary solutions that optimize or enhance raw generative model outputs. These include editing interfaces for text and images, control frameworks to align AI responses, filtering for sensitive content, synthetic data generation, and tools to augment data labeling and annotation processes. An effective generative Ai strategy likely incorporates capabilities from multiple vendors rather than relying solely on a single provider.
Identifying High-Potential Use Cases
The next step entails brainstorming and prioritizing potential enterprise use cases that can benefit from generative AI's unique capabilities. With possibilities spanning content creation, data enhancement, personalization, customer support, market research and even workplace automation, the range of options is endless. Avoid the temptation to boil the ocean. Generative AI remains an early-stage technology, so focusing investments on low stakes applications most aligned with business value and priorities tends to offer better returns than trying to revolutionize every process.
When evaluating use cases, analyzing the time and effort needed to achieve similar outcomes manually provides a yardstick for potential productivity improvements and cost savings. For instance, authoring a product catalog might take weeks of effort compared to minutes with an AI assistant. Similarly, tasks like answering customer emails that consume much employee time and frustrate clients often emerge as early wins. Processes that involve data labeling, searching documents in proprietary formats, or translating content to local languages also tend to benefit enormously.
Developing Custom Generative Models
While pre-trained foundations models provide a convenient starting point, customizing models trained on company-specific data can significantly enhance quality, relevance and alignment to business needs. Unlike generic and risky web-scraped training data, internally curated datasets allow teaching nuances around branding guidelines, product offerings, domain knowledge and other organizational sensitivities models won't pick up otherwise.
Constructing tailored datasets and model architectures requires data science capabilities, so this investment is only warranted for advanced use cases with sufficient scale. But the payoff can justify the effort. Where relevant, make model customization a plank of your generative AI strategy, either by building in-house capability or working with vendors offering customization services.
Implementing Rigorous Testing Standards
Testing rigor emerges as a critical determinant of success once generative models get deployed for business applications. Unlike traditional software that behaves predictably based on hand-coded logic, generative AI exhibits much more variability. Outputs depend enormously on prompts, which are challenging to construct reliably. Pre-launch testing helps catch issues, but problems often surface only upon live deployment.
Set up testing protocols that assess generative applications across relevant dimensions:
- Content quality - Does generated text or media meet intended style, depth and accuracy standards?
- Value alignment - Do responses demonstrate judgment consistent with corporate policies and ethics?
- Prompt efficacy - Do prompts reliably produce on-target outputs without needing excessive retries and rephrasing?
- Error handling - Does the system fail gracefully when given incorrect or out-of-scope inputs?
Leverage human review, simulations, and where possible, automated QA using diffusion models that detect flaws. Plan to continually evaluate performance across critical success metrics and application SLAs. Probe for objectionable or harmful content. Validation cannot end once models get initially cleared for release. Build ongoing monitoring and resilient feedback loops instead.
Instituting Ethical Guard Rails
Like any transformative technology, generative AI risks unintended negative consequences if deployed carelessly. Models can perpetuate harm by amplifying biases, spreading misinformation, plagiarizing content or infringing rights.
An ethical AI strategy minimizes downside risks through oversight mechanisms like advisory boards, harm assessments before launch, monitoring for toxic content post-release, and processes that allow removing objectionable system behaviors rapidly. Consider case-specific tradeoffs between free speech and harmful impacts. err on the side of caution by restricting generative applications for marketing or entertainment where risks outweigh benefits. Prioritize high-value domains like medical, scientific or analytical use cases.
Despite best intentions, avoids over promising on capabilities or safeguards initially. Be transparent about limitations and training processes. Seek diverse external input, test rigorously before launch, and observe outcomes closely once live. Course correct rapidly if issues emerge. Consider it your organization’s responsibility to ensure generative applications act professionally and avoid causing offense, even if end users primarily control final outputs. The foundational models themselves embed certain biases and flaws which vendors are working to address. So plan to layer guardrails customized to your use cases until core solutions mature further.
Instituting Continuous Improvement Loops
A robust generative AI strategy realizes capabilities today represent just the starting point. Like the internet and mobile apps, rapid iteration on applications, data, and oversight mechanisms will unlock increasing business value over time.
Plan upfront for accelerating improvement as models advance. Schedule capability upgrades to leverage new algorithms, enriched training datasets and platform features. Implement structured feedback channels to fix glitches, expand use case scope and optimize prompts at scale. Set up metrics dashboard and reviews to track enhancements quantitatively, demonstrate ROI and secure ongoing investment.
Equally importantly, put in place responsible disclosure channels for external stakeholders to report issues confidentially without fear of retribution. Such transparency and willingness to improve instills trust both internally and externally. Despite extensive testing before launch, problems will occur once live. Correct them without finger-pointing. View responsible disclosures as free and valuable input to enhance system quality proactively.
Key Takeaways
Developing an intentional strategy clarifies how generative AI can create business value responsibly, ensuring investments deliver maximum impact. Avoid ad-hoc experimentation or wholesale adoption without evaluating trade-offs. Prioritize use cases that play into existing strengths, even if starting small, rather than attempting transformational change immediately. Customize models to your data and needs where beneficial. Implement ethical guard rails appropriate to your domain. Focus on concrete business solutions rather than cutting-edge hype. Setup rigorous testing with resilience to failure built-in. Ultimately, plan for continuous responsible improvement as capabilities grow.
With prudent planning, generative AI can not just improve efficiencies but enable innovative applications you might not conceive today. But realize capabilities remain early-stage and imperfect. Set ambitions high but expectations appropriately modest to start. Build use cases iteratively, learn-as-you-go and ramp capability over time. With an adaptive and ethical strategy, generative AI can confer tremendous advantage. The recommendations outlined here aim to help develop such a strategy tailored to your unique business priorities and risk tolerances.