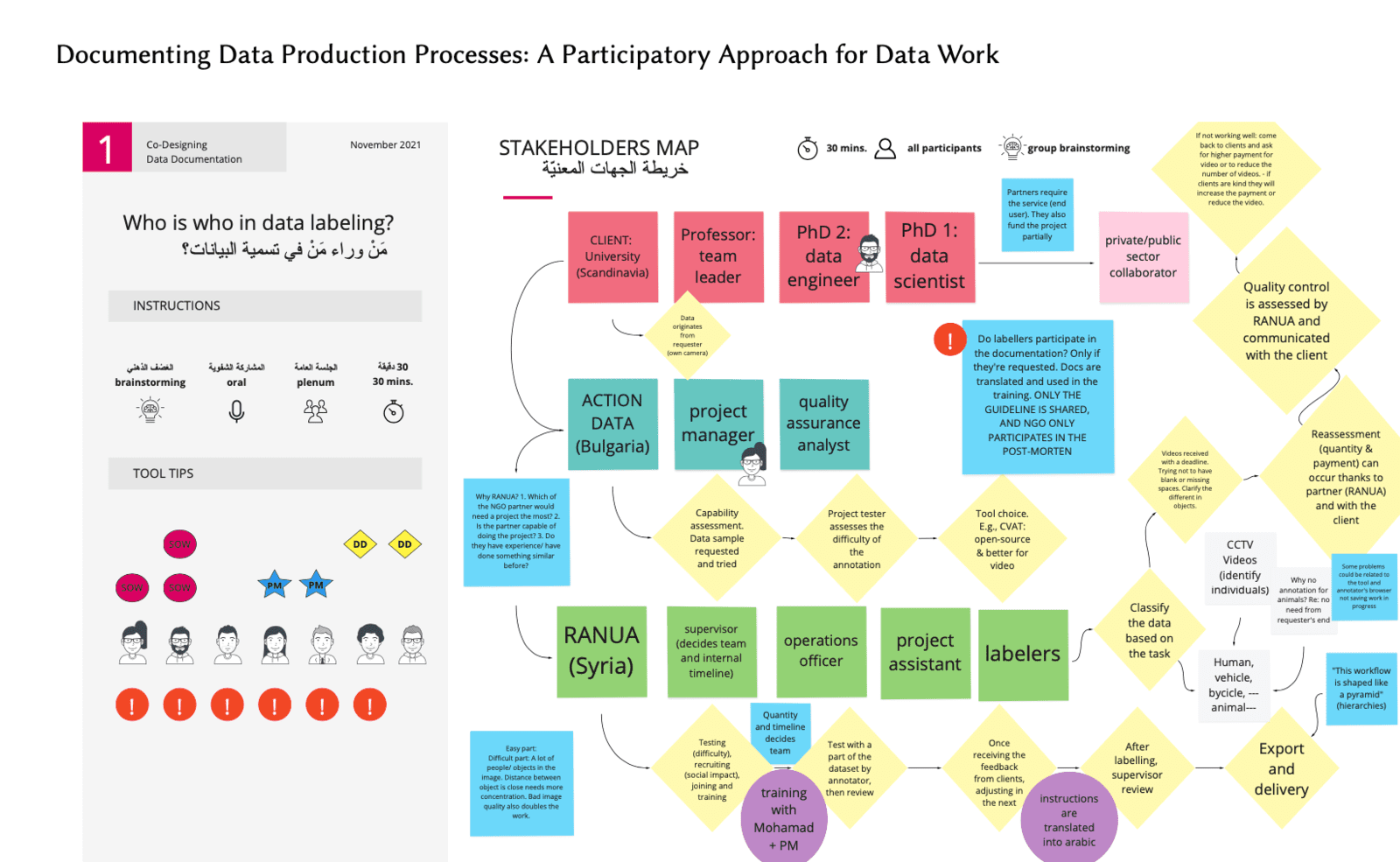
Machine learning (ML) systems are becoming increasingly prevalent, but few business leaders understand how the data used to train these systems is produced. A new study provides insights into the complex processes and people behind ML data work.
The study was conducted by researchers who partnered with two business process outsourcing (BPO) companies that employ human workers to collect and label data used for training machine learning systems. Through interviews and design workshops with data workers, managers, and clients, the researchers aimed to understand how documentation practices could be improved to capture the context and processes involved in producing ML training data.
Key findings:
- Data workers have limited visibility into the overall goals and uses of the projects they work on. Better documentation that explains the client, project timeline, and intended uses of the final dataset could improve coordination and workers’ sense of meaning.
- Data work involves collaboration between many geographically dispersed actors, but communication tends to be top-down. Enabling feedback loops in documentation could foster more participatory, iterative processes between clients, managers, and data workers.
- Data work is dynamic, but documentation practices are often static. Tools like revision histories and timeline tracking could better reflect the evolving nature of projects and datasets.
- Worker needs are not fully represented in current documentation. Including details like task instructions, payment terms, and ethical considerations could make documentation more worker-centric.
The researchers conclude that "data production documentation should travel across multiple stakeholders and facilitate their communication." Viewing documentation as a "boundary object" that adapts to diverse needs while maintaining integrity could enable more participatory and equitable data production processes.
Key implications for business leaders:
- Seek more visibility into how your ML training data is produced and provide input to vendors on documentation needs.
- Recognize and engage data workers as key collaborators, not just low-level laborers. Their feedback can improve dataset quality.
- Set documentation policies that make values like transparency, participation, and fairness explicit. This also mitigates business risks.
- Documentation supports coordination and accountability. Invest in tools and practices that reflect the dynamic reality of data work.
Milagros Miceli, Tianling Yang, Adriana Alvarado Garcia, Julian Posada, Sonja Mei Wang, Marc Pohl, Alex Hanna