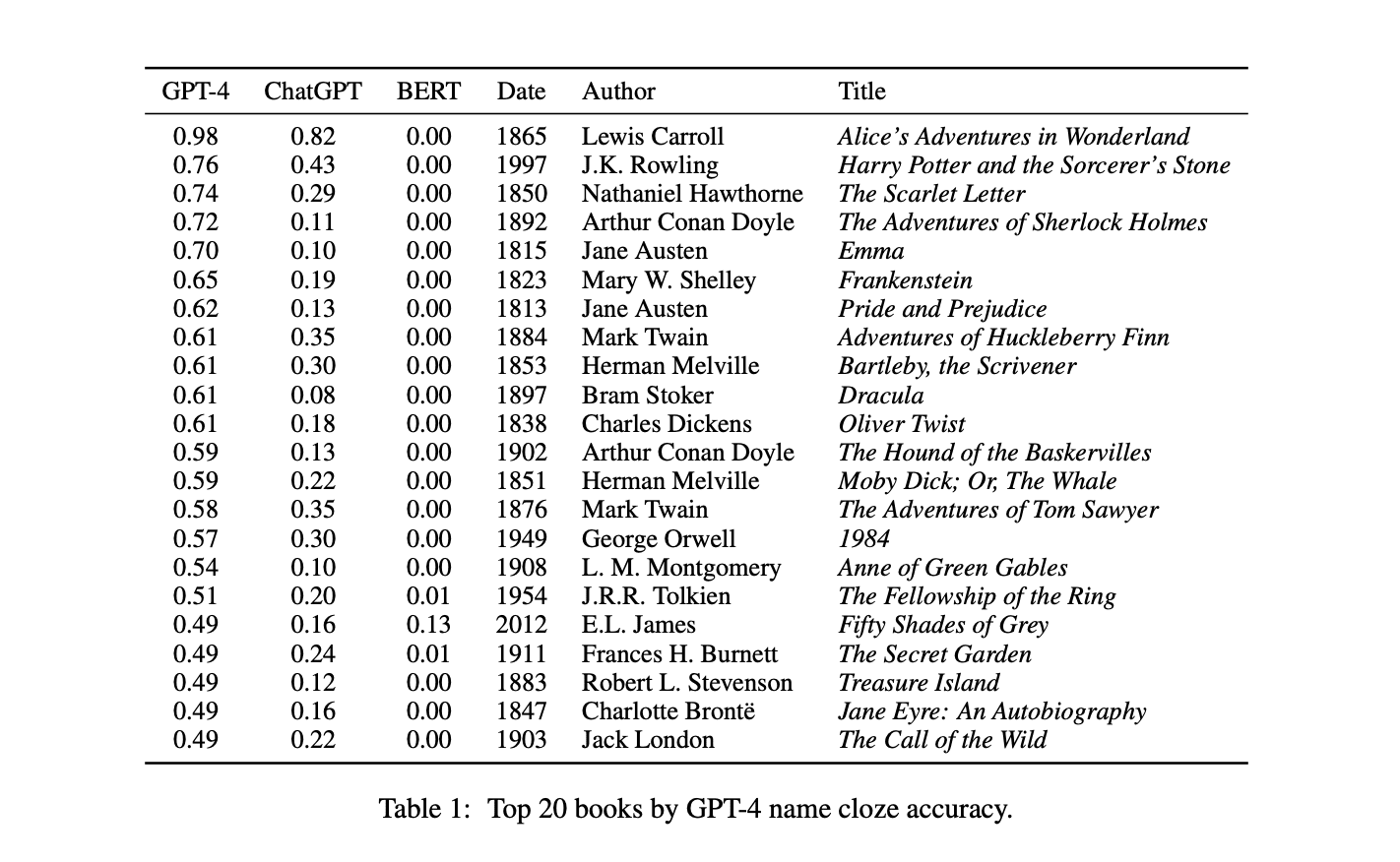
Artificial intelligence systems like ChatGPT and GPT-4 have demonstrated impressive language skills, holding fluent conversations and answering questions on virtually any topic. But their inner workings remain largely opaque to users. These systems are "black boxes" - we know little about what knowledge they actually contain.
New research from the University of California, Berkeley sheds light on one slice of these models' knowledge: which books they have "read" and memorized. The study uncovers systematic biases in what texts AI systems know most about, with implications for how we should evaluate them.
The researchers focused specifically on works of fiction. They selected a sample of 571 English novels published between 1749 and 2020, containing literary classics along with contemporary bestsellers and award winners. The sample spanned mystery, romance, and science fiction genres as well as global Anglophone and African American literature.
For each book, the team extracted short passages of 40-60 words containing a single character name - but with the name removed. For instance, a passage from Pride and Prejudice might read "______ entered the room and greeted her hosts warmly." Humans cannot guess the missing name from such brief context. But does the AI system know the name from having read the full book?
The researchers tested two systems, ChatGPT and GPT-4, by giving each passage and asking what single-word name belongs in the blank. The accuracy of each AI model on this challenging "cloze" task revealed what books it likely memorized.
The results illuminated clear biases. Both systems strongly favor science fiction and fantasy works like Lord of the Rings and Harry Potter over other genres. They excel at classic literature like Alice in Wonderland and Pride and Prejudice but fare poorly on modern award-winning diverse books. In short, they are more knowledgeable about popular texts.
What explains this imbalance? The researchers found it closely mirrors what's most duplicated across the internet. There is a strong correlation between AI accuracy on a book and the number of verbatim passages found through Google, Bing, and other sources. The models appear to "know" books in proportion to their web popularity.
This reliance on the internet has consequences. The study showed AI systems perform better at predicting a book's publication date and summarizing its passages when they have memorized the book. In other words, their reasoning is tied to memorization - causing disparities between popular versus niche texts.
These insights matter because AI systems like ChatGPT are increasingly used for applications like analyzing literature and human culture. If their knowledge comes largely from duplicated web text, focused on popular sci-fi and fantasy, how well can we trust their judgments about less mainstream books? Their skewed knowledge could propagate biases into downstream decisions.
The findings illustrate the challenges of opaque "black box" AI systems whose training data is secret. OpenAI, which created ChatGPT and GPT-4, has not revealed what texts were used to train them. This leaves us unable to fully assess their knowledge gaps.
The researchers argue we should instead push for more transparent, open-source AI systems whose training data is public knowledge. This allows us to better understand their strengths and weaknesses - illuminated through research like this study.
As AI models grow more capable and ubiquitous, it becomes only more important to peek inside their black boxes. Understanding what knowledge they contain helps ensure we build and apply them responsibly. Analyses of what systems like ChatGPT "know" about books mark an important step toward making AI more intelligible as it continues permeating our lives.
Sources: