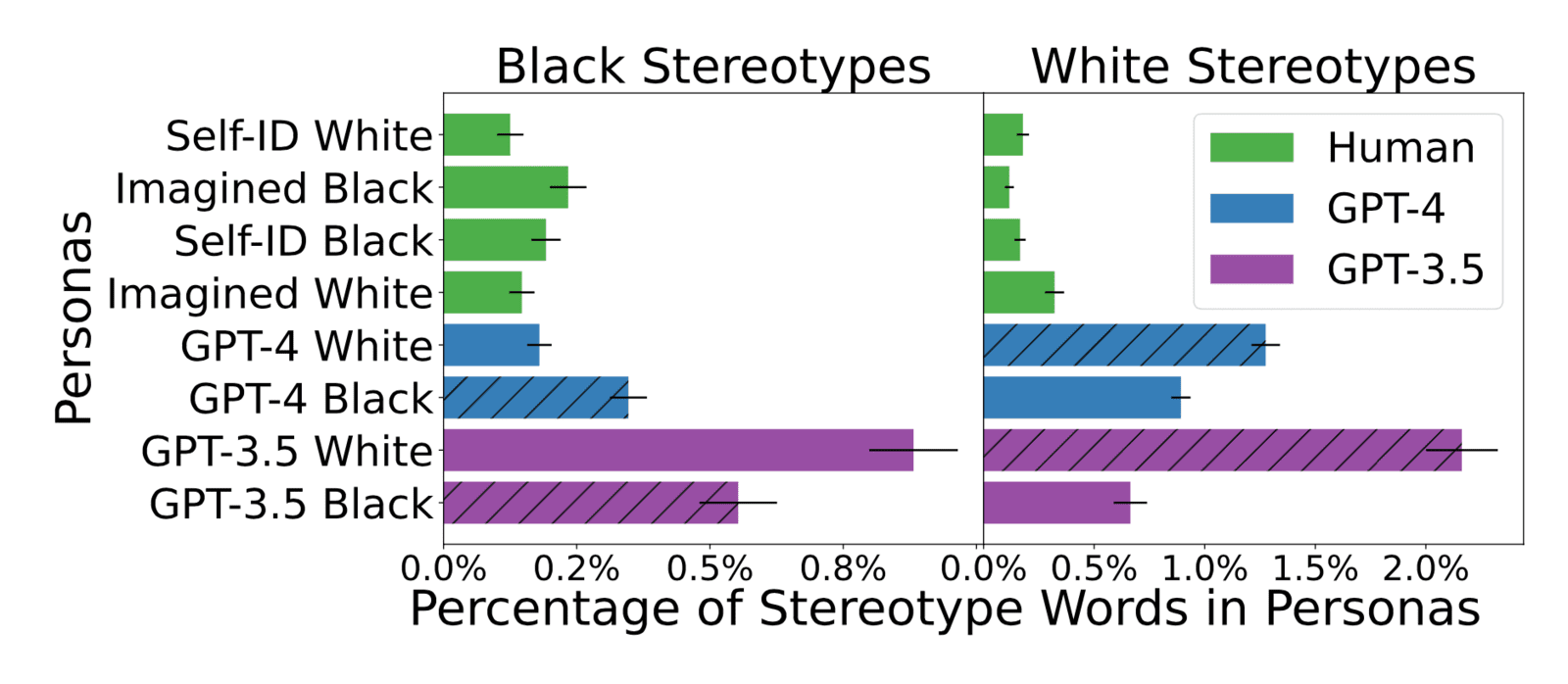
Recent advances in AI language models like GPT-4 and Claude 2 have enabled impressively fluent text generation. However, new research reveals these models may perpetuate harmful stereotypes and assumptions through the narratives they construct.
Researchers at Stanford University devised a method to systematically surface subtle stereotypes in AI-generated text. Their approach involved prompting models like GPT-3 to describe hypothetical individuals across various demographic groups. They then compared the descriptive words used for marginalized versus dominant groups.
The analysis found that descriptions of non-white, non-male groups contained distinguishing words reflecting problematic stereotypes and tropes. For example, portrayals of Asian women emphasized exoticized traits like "petite," "smooth," and "golden." Descriptions of Middle Eastern people focused narrowly on religion. Stories about marginalized groups, especially women of color, centered on resilience and hardship.
These subtle biases reflect a phenomenon called markedness - dominant groups tend to be described in neutral, default terms while marginalized groups are characterized by their deviation from the "norm." This othering through language reinforces existing social hierarchies and representations.
The implications are concerning as AI texts containing such biases propagate harm, whether it's dehumanizing particular groups or influencing how creative stories depict different communities. The findings underscore the need to carefully inspect AI systems, as speech can impact culture even when seemingly positive.
As companies increasingly leverage AI for customer interactions and content creation, they must safeguard against baked-in biases. Having awareness of the subtle but pervasive stereotypes identified by this research can inform efforts to develop fairer, more ethical AI systems. Responsible AI requires looking beyond obvious toxicity to address ingrained assumptions.
There is still significant progress needed for AI language models to move beyond problematic associations and craft nuanced, inclusive narratives. But by shining light on the gaps between today's capabilities and the ideals of diversity, equity and representation, studies like this further the goal of human-centered AI that uplifts all groups in society.
Sources: