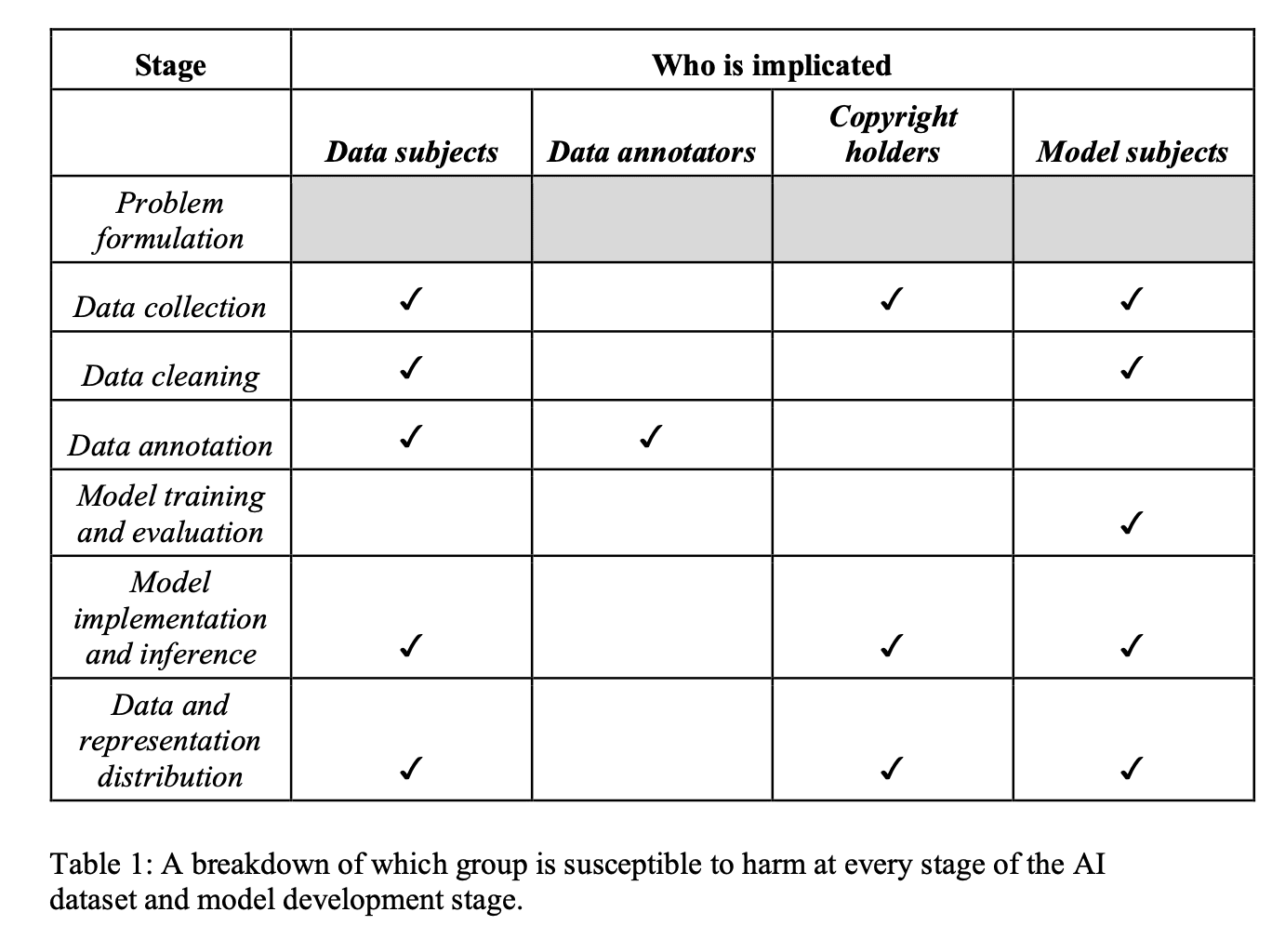
As artificial intelligence (AI) and machine learning technologies become more widely adopted across sectors, businesses must prioritize responsible and ethical implementation. A critical yet often overlooked area is how AI training datasets are constructed behind the scenes.
In a recent paper, researchers Mehtab Khan and Alex Hanna highlight the need for greater scrutiny, transparency, and accountability in how massive datasets for machine learning models are created. Their analysis proposes breaking the process into distinct stages and identifying affected individuals.
Legally Gray Areas Around Data Collection
The paper notes that current copyright law is ambiguous regarding reproducing large volumes of text, images, and other data for assembling AI training datasets through scraping websites and other sources. While fair use exemptions may technically apply in some cases, the legal boundaries are highly complex. This gray area allows widespread copying to build datasets, but concerning practices could still raise issues like copyright infringement.
Emerging Individual and Collective Privacy Concerns
Collecting data en masse, even from publicly available websites, risks violating the privacy of individuals included without their consent. But the paper emphasizes privacy issues extend beyond individual data collection. Biases and unfair representations affecting certain populations also clearly emerge when examining dataset design choices.
A Framework for the Entire Development Pipeline
To illuminate interconnected problems throughout the process, the researchers propose systematically analyzing each stage of assembling training datasets. This includes initial problem definition, data collection and cleaning, annotating examples, model building and evaluation, and distribution. Examining how different stakeholders are impacted at each phase can uncover ethical issues.
The paper argues this framework highlights where greater transparency, oversight, and accountability are most needed. It can also inform policies and self-regulation. Documenting datasets is one step, but comprehensive governance of the full development lifecycle is required for responsible AI.
Proactive Policies Needed
Understanding training data origins is no simple task but crucial for mitigating risks like biases, discrimination, and improper data usage. While technical teams focus on accuracy, leaders must invest in responsible data sourcing and stewardship. Establishing proactive policies and drawing on frameworks like the one outlined in this paper can lead to more ethical AI systems.
Sources:
The Subjects and Stages of AI Dataset Development: A Framework for Dataset Accountability
Mehtab Khan, Alex Hanna