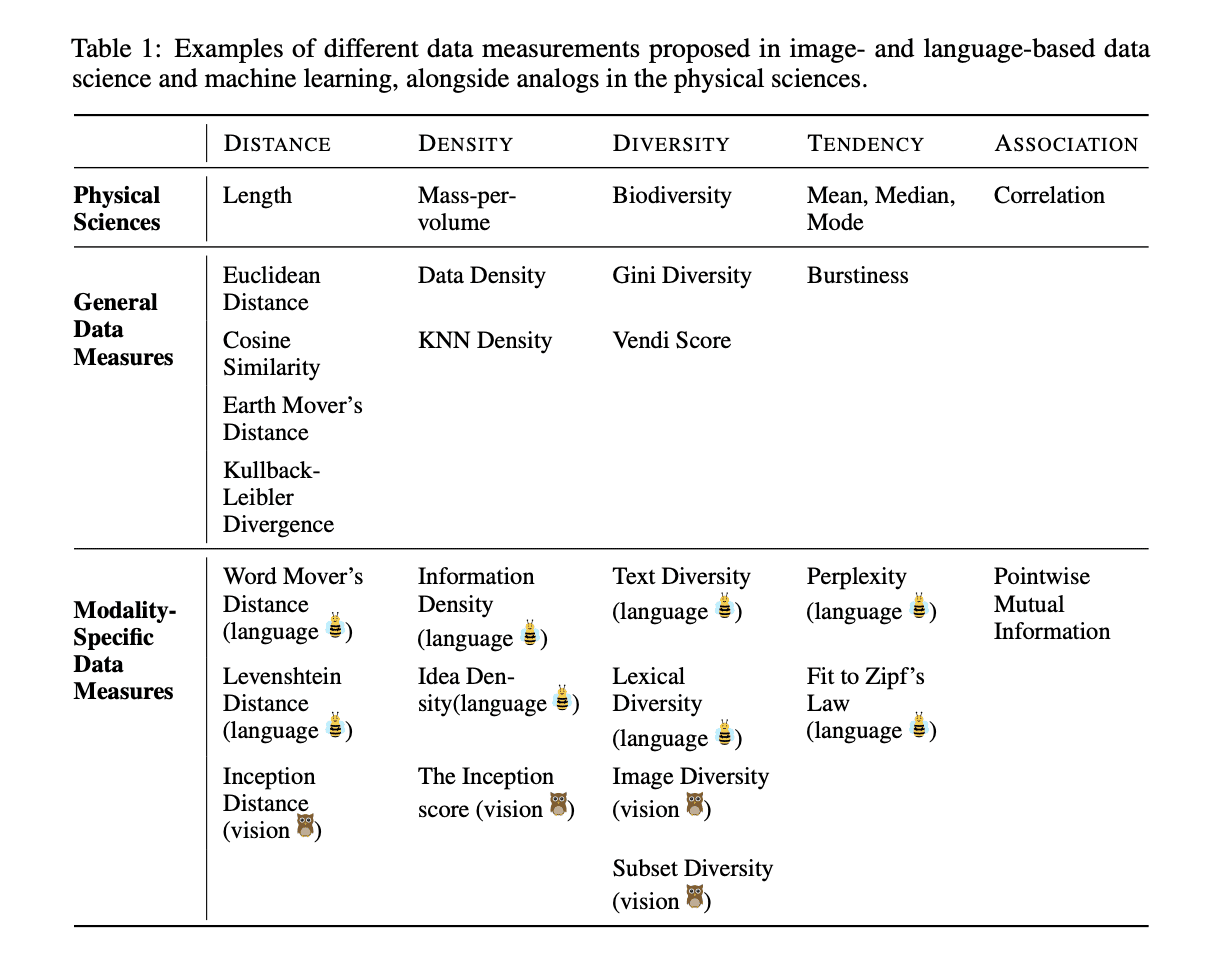
A new perspective paper argues for "measuring data" as a critical task to advance responsible AI development. Just as physical objects can be measured, data used to train AI systems should also be quantitatively analyzed to understand its composition.
The authors propose formalizing data measurement as a core research area. They categorize different measurement types like distance, density, and diversity. Distance metrics like Euclidean distance capture similarities in the data. Density reflects how densely certain concepts are represented. Diversity measures heterogeneity.
The paper summarizes measurements proposed across computer vision, natural language processing, and general data science. For instance, perplexity scores indicate how predictable text data is. Image density models visual patterns. Word mover's distance compares document similarity based on word embeddings.
The authors argue precise data measurements will let practitioners better curate datasets and understand what models learn. Characterizing training data is crucial for responsible AI. Metrics can reveal underrepresentation of groups or embed problematic biases.
For business leaders deploying AI, this paper provides a framework to evaluate data rigorously before training models. Combining multiple measurements will enable deeply understanding datasets. Just as standardized physical measurements enabled advances in science, quantifying data properties will drive progress in AI. Insisting models be trained on measured data is vital for ethical AI.
Sources: