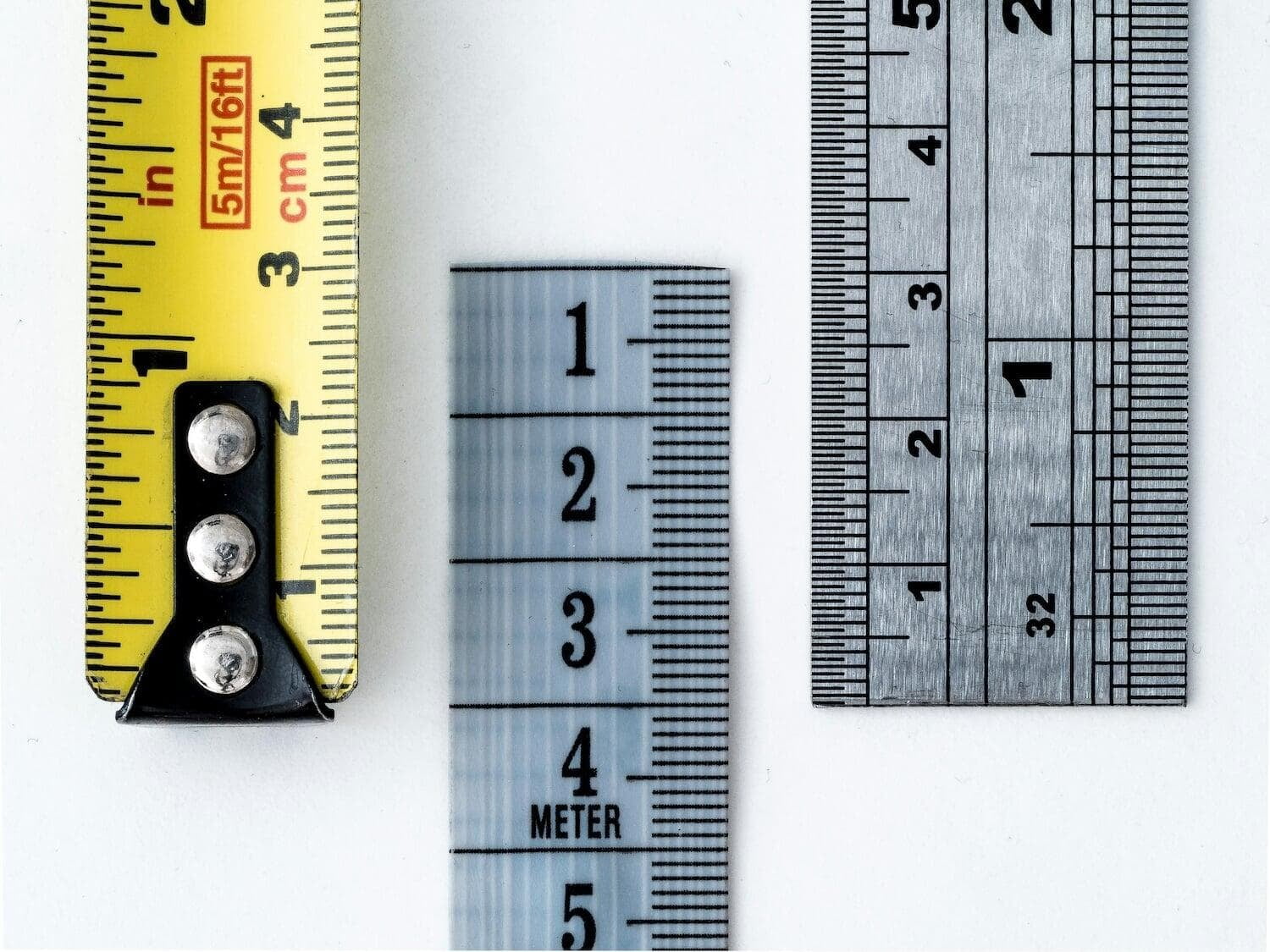
Artificial intelligence (AI) has made remarkable strides in recent years, with AI systems now achieving impressive performance on a variety of tasks, from image recognition to language understanding. These advancements have been largely driven by the development of powerful machine learning algorithms, coupled with the availability of vast amounts of training data and computational resources.
However, as AI continues to progress, it is crucial for business leaders to understand the limitations and potential pitfalls of current approaches to measuring AI capabilities. A position paper by Raji et al. offers a compelling critique of popular AI benchmarks, arguing that they are often misleading and fail to capture meaningful progress towards general intelligence. This critique is further echoed in a recent TechCrunch article by Kyle Wiggers, which highlights the disconnect between AI benchmarks and real-world applications.
The Allure of "General" AI Benchmarks
Two of the most widely cited benchmarks in AI are ImageNet, used for evaluating image recognition systems, and GLUE (General Language Understanding Evaluation), used for assessing natural language processing models. These benchmarks have taken on an outsized role in the AI community, with performance on these tasks often seen as indicative of progress towards general AI capabilities.
The appeal of these benchmarks is understandable. They offer a standardized way to compare different AI systems and track improvements over time. Moreover, the tasks they encompass, such as identifying objects in images or understanding the meaning of sentences, seem to capture essential aspects of intelligence that humans excel at.
However, as Raji et al. point out, these benchmarks are far from perfect measures of general intelligence. In fact, they argue, the focus on achieving state-of-the-art performance on these narrow tasks has distorted the priorities of the AI research community and led to an overemphasis on benchmark-chasing at the expense of more meaningful progress.
The Limitations of Current Benchmarks
One of the key criticisms leveled by Raji et al. is that the tasks included in popular AI benchmarks are often arbitrary and not systematically chosen to represent general capabilities. They compare this to a fictional children's story about a museum claiming to contain "everything in the whole wide world," but which actually just contains a haphazard collection of random objects.
Similarly, the authors argue, benchmarks like ImageNet and GLUE are composed of a relatively narrow and idiosyncratic set of tasks that hardly capture the full range of intelligent behaviors. Impressive performance on these tasks is often taken as evidence of general intelligence, when in reality it may simply reflect a system's ability to exploit specific patterns or statistical regularities present in the training data.
The TechCrunch article by Wiggers further underscores this point, noting that many of the most commonly used benchmarks for chatbot-powering AI models, such as GPQA ("A Graduate-Level Google-Proof Q&A Benchmark"), contain questions that are far removed from the everyday tasks most people use these models for, such as responding to emails or writing cover letters. As Jesse Dodge, a scientist at the Allen Institute for AI, puts it, "Benchmarks are typically static and narrowly focused on evaluating a single capability, like a model's factuality in a single domain, or its ability to solve mathematical reasoning multiple choice questions."
Another issue highlighted in both the Raji et al. paper and the TechCrunch article is the presence of errors and flaws in some widely used benchmarks. For example, an analysis of the HellaSwag benchmark, designed to evaluate commonsense reasoning in AI models, found that more than a third of the test questions contained typos and nonsensical writing. Similarly, the MMLU benchmark, which has been touted by vendors like Google, OpenAI, and Anthropic as evidence of their models' logical reasoning abilities, contains questions that can be solved through mere memorization rather than genuine understanding.
As David Widder, a postdoctoral researcher at Cornell studying AI and ethics, notes in the TechCrunch article, "A model can't [reason through and solve new and complex problems] either" just because it performs well on benchmarks like MMLU. Instead, he argues, these benchmarks often test a model's ability to "memoriz[e] and associat[e] two keywords together" rather than truly understand causal mechanisms.
Key Takeaways for Business Leaders
Given the limitations and potential misleading nature of current AI benchmarks, what should business leaders keep in mind when evaluating AI technologies? Here are some key takeaways from the Raji et al. paper and the TechCrunch article:
- Be skeptical of grand claims about AI systems achieving human-level or superhuman intelligence based solely on benchmark performance. As both sources emphasize, impressive results on specific benchmarks do not necessarily translate to general intelligence or robustness in real-world deployments.
- When evaluating AI vendors or technologies, look beyond top-line benchmark numbers. Ask detailed questions about the specific capabilities and limitations of the system, and how it has been tested on tasks and datasets relevant to your business needs.
- Encourage a culture of rigorous, multifaceted evaluation within your organization's AI initiatives. Rather than focusing solely on chasing state-of-the-art benchmark results, prioritize detailed error analysis, bias auditing, and stress testing across a diverse range of scenarios.
- Support research and development efforts aimed at creating more meaningful and comprehensive benchmarks tied to real-world applications. This could include developing industry-specific datasets and evaluation protocols that better reflect the challenges and requirements of your business domain.
- Foster an AI research culture that values creativity, diversity of thought, and long-term progress over short-term benchmark wins. Encourage your teams to explore novel architectures and approaches, even if they may not immediately yield chart-topping results.
Looking Ahead: Improving AI Benchmarks
Both the Raji et al. paper and the TechCrunch article offer some suggestions for improving the current state of AI benchmarks. One key idea is to incorporate more human evaluation alongside automated benchmarks. As Jesse Dodge suggests in the TechCrunch piece, "The right path forward, here, is a combination of evaluation benchmarks with human evaluation—prompting a model with a real user query and then hiring a person to rate how good the response is."
David Widder, on the other hand, is less optimistic about the potential for improving existing benchmarks. Instead, he argues that AI evaluation should focus more on the downstream impacts of these models and whether those impacts align with the goals and values of the people affected by them. "I'd ask which specific contextual goals we want AI models to be able to be used for," he says, "and evaluate whether they'd be—or are— successful in such contexts."
As AI continues to advance and become more deeply integrated into business operations, it is crucial for leaders to have a nuanced understanding of the technologies' strengths and limitations. By looking beyond simplistic benchmark results and embracing a more holistic and rigorous approach to AI evaluation, organizations can make more informed decisions and unlock the true potential of artificial intelligence while mitigating its risks and pitfalls.
Footnotes:
- "AI and the Everything in the Whole Wide World Benchmark" by Inioluwa Deborah Raji, Emily M. Bender, Amandalynne Paullada, Emily Denton, and Alex Hanna
- "Why most AI benchmarks tell us so little" by Kyle Wiggers for TechCrunch
Photo by William Warby on Unsplash