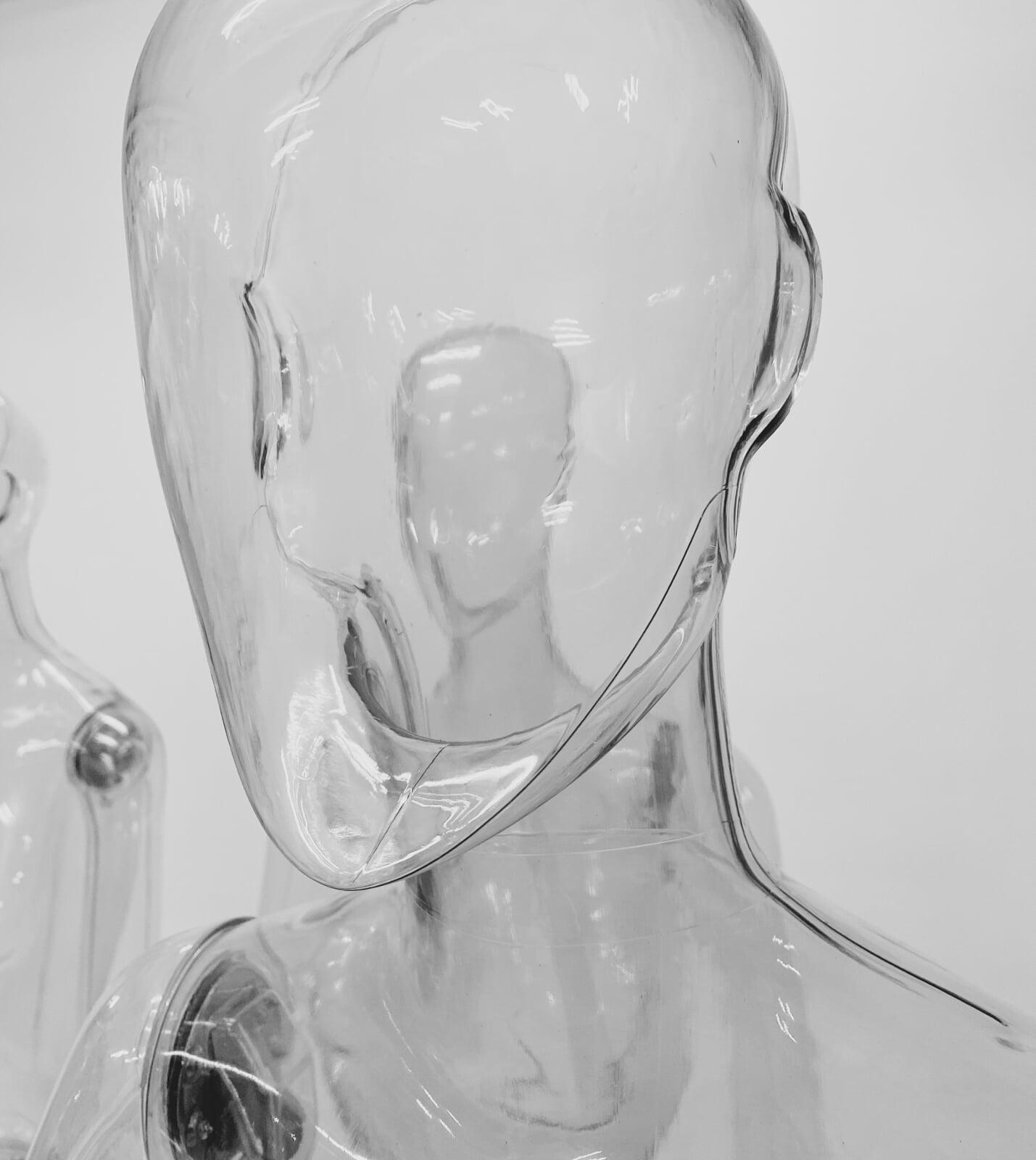
A recent critique calls into question a prominent AI transparency benchmark, illustrating the challenges in evaluating something as complex as transparency.
Earlier this month, we reported that researchers at Stanford University released the Foundation Model Transparency Index, an effort to assess and score leading AI systems on 100 metrics related to transparency. The index aimed to provide an empirical view into the often opaque development of artificial intelligence.
However, the index has faced sharp criticism for misrepresenting transparency and introducing methodological flaws. In a detailed rebuttal titled "How the Foundation Model Transparency Index Distorts Transparency," researchers affiliated with the nonprofit EleutherAI argue the index distorts more than it reveals.
The critique makes several core assertions:
- The index conflates transparency and corporate responsibility. Many of the 100 metrics relate more to issues like moderation policies and terms of service versus research reproducibility.
- Openly released models score poorly despite transparency being a goal. Models that prioritize releasing datasets, code, and weights score low since the index underweights these factors.
- Questions introduce bias against certain projects. Many metrics favor commercial services over research efforts and introduce unreasonable requirements like disclosing salaries.
- Factual errors and misrepresentation are common. Multiple models are docked points incorrectly due to misinterpreting or overlooking documentation.
- Aggregate scoring obscures nuance. Collapsing 100 complex metrics into a single 0-100 score encourages gaming and misuse of the ratings.
The critique argues the index will likely lead to "transparency theater" where companies generate documentation solely to boost scores without meaningfully improving openness. Researchers involved contend the index conflicts with its own stated goals around enabling ecosystem health.
The issues raised serve as an important reminder that quantifying something as nuanced as transparency is enormously challenging. Even well-intentioned measurement risks reinforcing bias, oversimplification, and unintended incentives.
For business leaders, this debate underscores the need for diligence in evaluating AI systems. Metrics like the transparency index may provide a useful starting point but require close scrutiny themselves. When assessing responsible AI practices, metrics should be just one input into a holistic process also accounting for factors like direct audits, benchmark tests, and qualitative reviews.
The path towards genuinely transparent and trustworthy AI will require sustained coordination between companies, researchers, regulators, and civil society. For now, business leaders would be wise to approach AI transparency rankings and research with a critical eye.