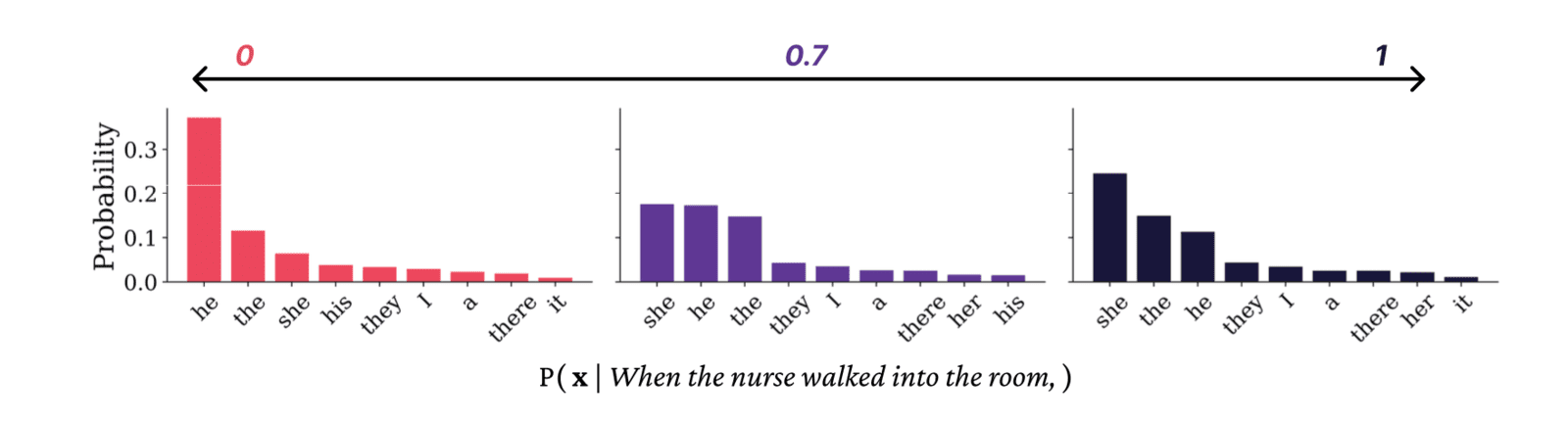
Artificial intelligence has made great strides in recent years, especially in natural language processing. Systems like ChatGPT and Claude can now hold impressively human-like conversations. However, a major limitation of these AI language models is that they operate like a black box - their internal workings are complex and opaque.
Researchers at Stanford have proposed a new AI architecture called Backpack that aims to fix this problem. Backpack models have an internal structure that is more interpretable and controllable compared to existing models like BERT and GPT-3.
Here's an analogy to understand how Backpack works:
Think of words as Lego blocks. Each block can be connected to other blocks in many ways to build something. Existing AI models are like throwing all the Lego pieces together in a pile - there are endless ways to combine them, but you can't understand or control the resulting structure.
A Backpack model is more like having clearly labeled Lego pieces in different bags. For each word, there are "sense vectors" that represent its different meanings and uses. When the model sees a word in a sentence, it decides which sense vectors to pull out of the bag to understand and predict that usage.
This structure offers two key benefits:
- Interpretability: We can inspect the different sense vectors for a word and understand what aspects of meaning they represent. This is like looking inside the bags to see the different kinds of Lego pieces.
- Control: We can directly edit the sense vectors to change the model's behavior. For example, reducing a gender-biased sense vector for the word "nurse" can reduce sexist outputs. This is like removing certain Lego pieces from a bag to change what can be built with it.
In initial tests, Backpack models matched the performance of existing models like GPT-2 while offering far more transparency. Researchers were able to do things like swap associations (so "MacBook" predicts "HP" instead of "Apple") and reduce gender bias in occupations.
The inventors stress that Backpack is still early stage research. The approach needs to be scaled up and tested across different languages and applications. But it represents an exciting step towards AI systems that are not black boxes. Instead of blindly trusting model outputs, users can interpret why it behaves in certain ways and directly edit its knowledge.
As AI becomes more powerful and ubiquitous in products and services, retaining human agency is crucial. Approaches like Backpack could make future AI not only smarter but easier to understand and actively improve. Business leaders should track developments in interpretable AI closely, as it is an important competitive differentiator down the line.
Source: