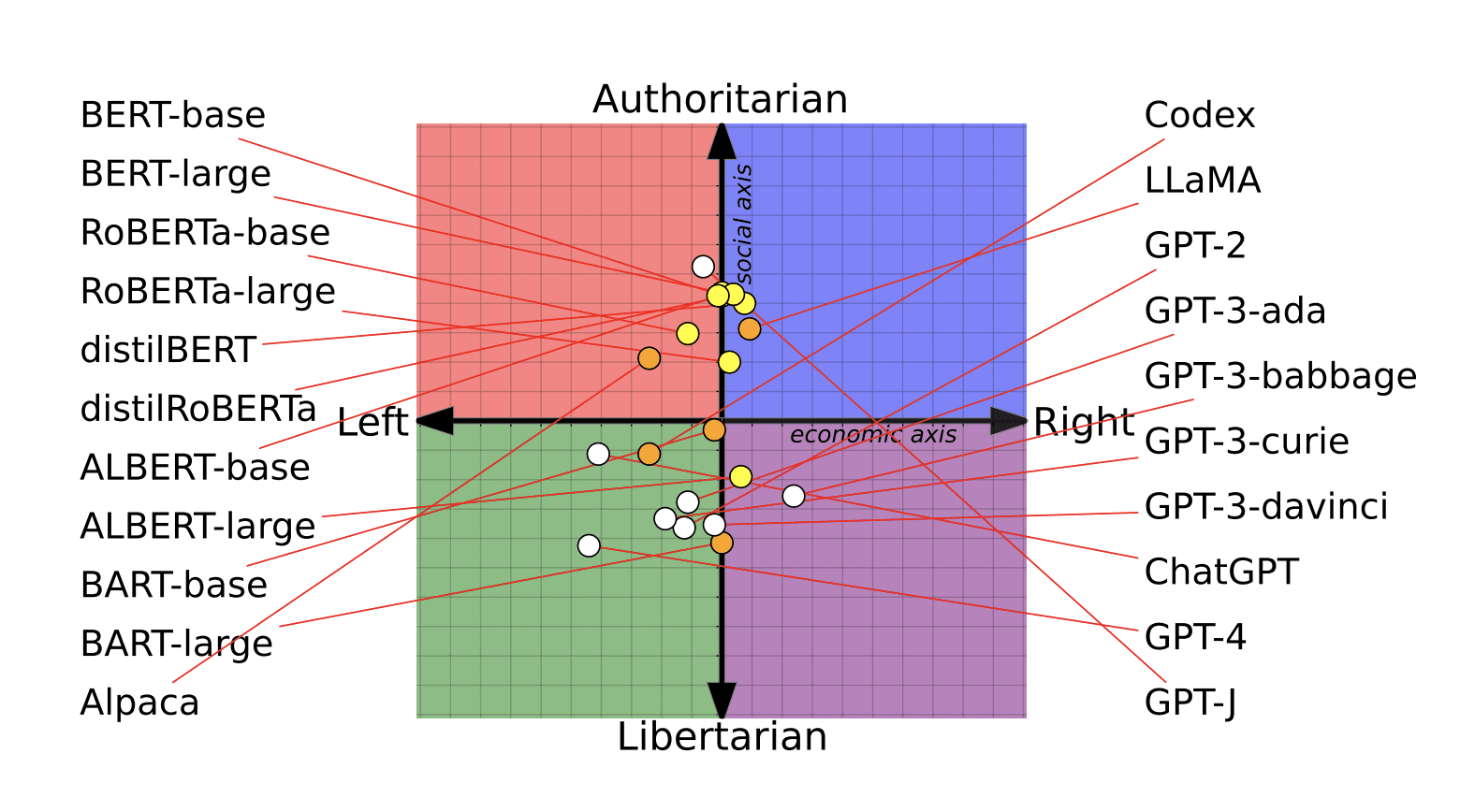
Recent advances in AI, especially large language models like GPT-4 and Claude 2, have unlocked new capabilities in generating text and speech. However, these models are still "sponges" that absorb patterns, including potential societal biases, from their training data. A new study from the University of Washington digs into an important question - can political bias in the data propagate to the models and affect downstream decisions? Their findings highlight risks that businesses should be aware of when deploying these technologies.
The researchers focused on political leanings across two dimensions - social values (liberal vs conservative) and economic values (left vs right). First, they evaluated the inherent biases of 14 major language models by analyzing their responses to statements from a standard political compass test. The models occupied a range of positions across the political spectrum, with BERT variants being more conservative and GPT models more libertarian.
Next, they examined if further pretraining these models on partisan news and social media corpora leads them to shift their political stances. The results show that left-leaning data induces liberal shifts, while right-leaning data causes conservative shifts. However, the overall shifts were small, suggesting inherent biases persist.
Finally, they tested if these biased models perform differently on social-impact tasks like hate speech and misinformation detection. While overall performance was similar, models exhibited double standards - left-leaning ones were more sensitive to offensive speech targeting minorities but overlooked attacks on dominant groups. Right-leaning models showed the opposite.
For business leaders, these findings highlight risks of unintended bias and unfairness creeping into AI systems built on large language models. While some bias is inevitable given the training data, being aware of its extent and impact can help inform ethical AI practices. Companies should proactively probe for biases, use diverse evaluation datasets, and leverage different perspectives through ensemble approaches.
Tracking bias from data to models to decisions is vital for ensuring AI transparency and accountability.
Source: